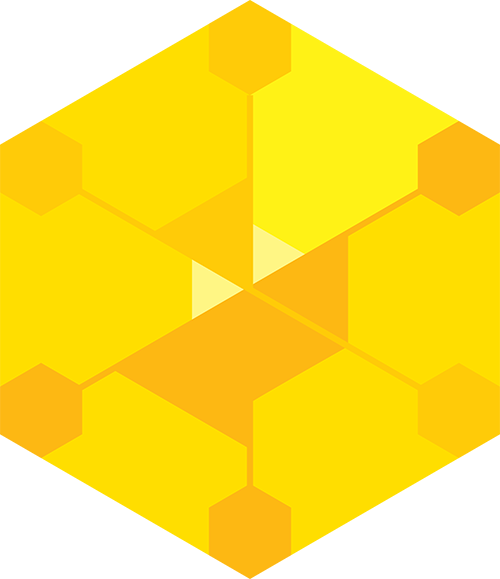
Our big data analytical platform provides solutions addressing challenges in various regulatory, healthcare and biomedical research areas. HIVE has dozens of algorithms and hundred+ pipelines addressing challenges from the variety of computational healthcare and biomedical research areas. We also conduct study specific analyses whenever required
AI/ML analytics for clinical research
Aside from image analysis using automated algorithms, we use AI/ML methods whenever there is need to consider complex interactions between predictors and need to identify patient populations among whom the treatment works best. The information may help clinicians understand population-specific treatment effect better and assist with clinical decision making.
Distributed analytics
We have developed methodologies that enable the distributed analysis of national and international data sources. When using this approach, a standardized data extraction program is implemented and distributed to participating data partners. Each partner then only runs the program on their data with no new analytic effort. The programs generate completely de-identified data summaries that are sent back to the data coordinating center. Data are then combined using multivariable hierarchical models to evaluate comparative outcomes of therapeutics including analyses of effects in all pre-specified subgroups.
Data linkage tools
To advance linkages between clinical trials, registries and routinely available data sources (e.g. claims and administrative data), our partners are continuously developing and refining linkage algorithms. We have tools for anonymous linkages to augment the research capacities of registries and trials by bringing together trials, registries, claims data, and electronic health records. Our data linkages with indirect identifiers are reliable with high sensitivity and accuracy. The linkages are often very cost-effective way to obtain long-term outcomes and enable long-term surveillance for technologies.
Natural Language processing
Natural language processing (NLP) is incorporated into select HIVE automated algorithms. We have well developed algorithms for analyses of passive reporting systems maintained by FDA such as Vaccine Adverse Event Reporting System (VAERS) and Manufacturer and User Facility Device Experience (MAUDE). NLP is a valuable method that can be used to parse unstructured text data and extract information from adverse event reporting systems, medical notes, radiology reports. NLP can be efficient and labor saving for processing large amount of text data. We are currently developing additional algorithms for NLP use that will help advance the registries and EHRs based surveillance systems. We have a federally funded study focusing on cancer data collection to enhance efficiency and sustainability of research networks in cancer surgery and interventions.
Claims data analytics
In collaboration with our partners, we have global leadership in using administrative databases for technology/device safety and effectiveness evaluation. When working with these data sources clinical knowledge and using clinical and scientific judgment is critical due to limitations of the data. Asking answerable clinical questions requires not only collaboration of clinicians with methodologists but also continuously training methodologists in medicine and surgery and clinicians in methodology. We have leading experts with decades of expertise and our core experts have done over 250 studies using administrative databases in the past 5 years alone. We recently completed the transition from ICD 9 to ICD 10 which is critical for database research in the modern era. Many conditions and events have been defined and translated to ICD 10 algorithms to support research. We conduct validation studies of new coding definitions to verify their clinical accuracy.
Active surveillance
The concept of active surveillance includes organization of entire data system based on real world evidence. However, it is important to have analytic tools that enable automation in analyses using ‘plug and play’ algorithms. To support this methodology including the need for long-term surveillance HIVE has developed flexible tools to provide users with timely and comprehensive evaluations of medical technology safety signals. There are tools using mobile platform for direct data collection and also tools for continuous data feeds from registries or other real world data systems. Our methodology is robust and we continuously improve it. We have incorporated all known tools for outlier detection and partnered with leading experts for including risk adjusted automated surveillance algorithms such as DELTA system.
Meta-analyses
We conduct comprehensive evidence syntheses/meta-analyses after thorough evaluation of risk of bias and heterogeneity of data. Using clinical and scientific judgement is critical for conducting meta-analyses. Our team contributed and advised the stakeholders in many high-profile meta-analyses related to technology safety and effectiveness. Choosing the right model is critical and may have a major impact on estimate of the effect. Hence, we carefully consider the models (e.g. fixed vs random effect) and use of advanced methods such as network meta-analyses, distributed data analyses/pooling and Bayesian approaches. We have conducted evidence syntheses using both published data and individual patient data (IPD) from clinal trials. Whenever appropriate, we conduct cross-design evidence syntheses to take advantage of all available information.